AI-Engine for Optimizing Mixed-Fleet Transit Operations
Funded in part by the Department of Energy under Award DE-EE0009212
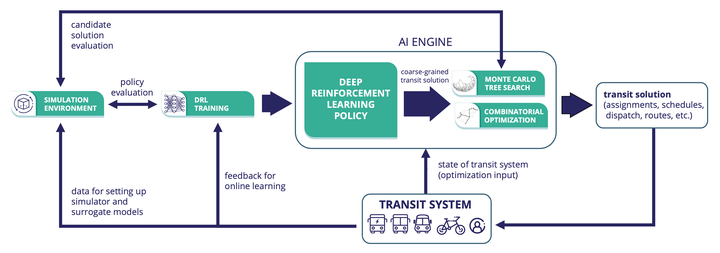
In every public transit system, a trade-off has to be made between concentrating service into very useful routes that serve large numbers of people and spreading service out to ensure that people everywhere have access to at least some service. Improving the efficiency of an existing system while enhancing service in terms of both usefulness and coverage presents considerable challenges. These challenges to operational efficiency are exacerbated by the requirement to provide complementary paratransit services, which are typically characterized by very low efficiency (energy per passenger per mile) and attendant high cost of operation. Our vision is to address these challenges by combining the complementary advantages of fixed- and dynamic-route transit services and seamlessly integrating them. We focus on the following objectives: minimizing energy used per passenger per mile, minimizing passenger wait and trip times, maximizing service coverage, and maximizing the percentage of daily trips serviced by transit. To explore this complex decision space, we will design, implement, and evaluate an artificial intelligence engine, which will enable agencies with mixed-vehicle fleets (EVs, ICEVs, etc.) to operate integrated fixed-dynamic transit services that maximize energy efficiency and make transit more accessible.
This project will bring together a team consisting of people with complementary background in transit operations, transit optimization, simulations, and artificial intelligence. This team will address the above challenges using state-of-the-art artificial intelligence, machine learning, and data-driven optimization techniques. Deep reinforcement learning and Monte-Carlo tree search will form the core of our real-time operational optimization, which will be supported by data-driven optimization for offline planning and by machine learning techniques for predicting transit demand, maintenance requirements, and traffic conditions.
The success of transit optimization and increased ridership depend on the acceptance of the public. Therefore, we are going to develop a novel methodology based on social relational networks to maximize our community outreach. We will augment these methods by providing affordable and complete transit subscription packages and a guidance app leveraged by CARTA’s unique role in managing transit, parking, bike share, and EV car share to support integrated subscription-based access to multiple modes of transportation. To mitigate the risk of operationalization, we will leverage Siemens’ Railigent platform, which provides the capability to manage large-scale geo-spatial data in the cloud and to generate comparative analytics. This partnership also ensures that the software developed here will be of a high technical readiness level and will be provided with required maintenance support to other agencies that face similar problems.
The impact of this project will be immense. The project provides mechanisms to improve transit accessibility with a projected increase from 41% to 73% of residents within ¼ mile of frequent transit service which will reduce personal-vehicle trips taken by those residents, significantly decreasing the energy used for transportation at the city level. The project will also reduce the per passenger per mile energy use and operating costs of the public transit service through operational optimization.